Designing an AI Signal Delivery System for VC Decision-Makers
- Excel Media Works
- May 6
- 3 min read
Updated: 4 days ago
How I balanced relevance, timeliness, and trust in an AI-driven product
When I joined a data-driven investment platform focused on helping VCs make faster, more confident funding decisions, one of our core challenges was this: How do we deliver AI-generated signals that decision-makers can actually trust and act on?
In this post, I’ll walk through how I approached the product thinking, design trade-offs, and implementation strategy behind our AI signal delivery system — a key feature that shaped investor behavior on the platform.
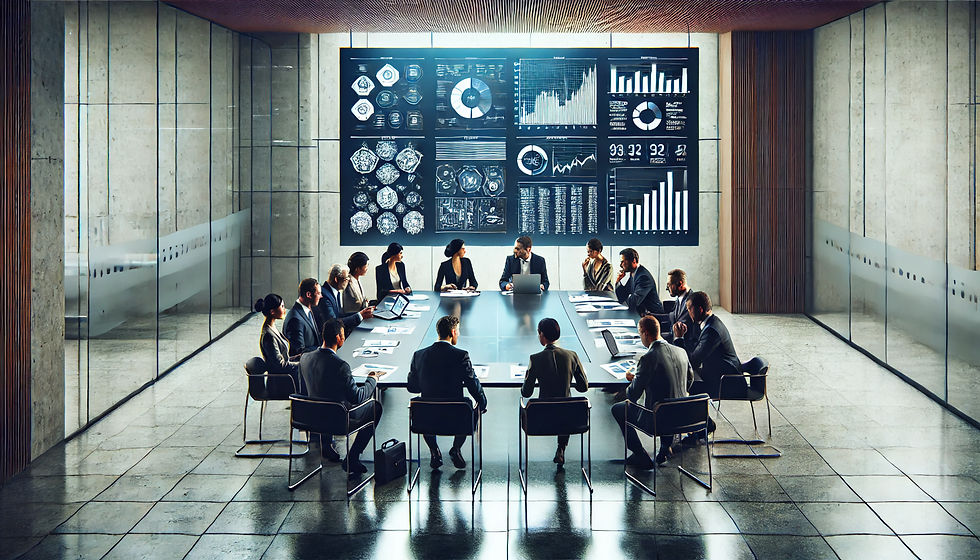
The Problem: Too Much Data, Not Enough Signal
Venture capitalists are flooded with data — financials, news, team performance, product usage, competitor moves. While our platform had access to alternative data sources and machine learning models, surfacing every insight would only lead to noise.
What they needed instead was:
The right signal, at
The right time, with
The right context to act on it.
And that’s what I set out to build.
My Approach: Product Strategy Meets Responsible AI
As a Product Manager, I structured this problem into four key areas:
1. Define What Counts as a “Signal”
I partnered with data scientists and domain experts to narrow down high-leverage events, such as:
Sudden user growth in a private startup
Strategic hires in product or engineering
Regulatory changes impacting specific sectors
Sentiment shifts in founder coverage
Not every data point was a signal. We built a framework for classifying actionable events vs informational noise.
2. Prioritize Use Cases, Not Features
Rather than building a generic “AI feed,” we asked:
When do VCs actually open the platform?
What questions are they trying to answer in that moment?
This informed both the UX design and the signal ranking logic. For example:
A VC focused on B2B SaaS Series B rounds got a different stream than one tracking fintech pre-seed deals.
We added custom watchlists to tune relevance and reduce fatigue.
3. Human + Machine Collaboration
We didn’t expect investors to blindly trust GenAI summaries. So we layered transparency into the design:
Signals included source links, AI confidence scores, and rationale behind the alert.
Users could provide feedback (“useful,” “irrelevant,” “already known”) which fed back into the model weighting.
This helped build trust in the system while also improving our models iteratively.
4. Speed + Delivery Mechanism
We tested different channels (in-app, email, Slack) and found that Slack-based delivery of urgent signals drove the fastest engagement, especially for partner teams.
We also had to balance real-time vs digest delivery. Our final setup used:
Instant alerts for critical events
Daily digests for pattern recognition
Weekly “What You Missed” summaries with GenAI commentary
Outcome: From Feature to Differentiator
Within one quarter of launch:
Signal engagement rose by 47%
Platform retention improved in our core VC cohort
Several users cited the feature in renewal and expansion calls
More importantly, we shifted our positioning from a data warehouse to a decision-support layer — powered by AI, but designed for human judgment.
Reflection: What This Taught Me About Product + GenAI
This project reinforced something I believe deeply: AI should serve as a lens, not a floodlight.
As a PM, my role wasn’t to show off what the model could do — it was to make sure what it did was relevant, usable, and aligned with user intent.
I also learned the importance of explainability and incremental trust in AI systems. You don't launch a feature; you earn adoption.
If you’re building GenAI into a decision-support product or want to discuss signal design, feel free to connect.
Comentarios