Metrics That Matter: Lessons from the Frontlines of AI Product Management
- Excel Media Works
- May 6
- 2 min read
Updated: 3 days ago
When I first transitioned into AI product management, I did what most of us do — I reached for the comfort zone of metrics. Accuracy. Precision. Recall. It felt familiar, measurable, “safe.” But as the months went by, something didn’t sit right.
We’d celebrate a model that had a 92% accuracy rate, only to discover that users didn’t trust it. Or worse — they weren't even using it.
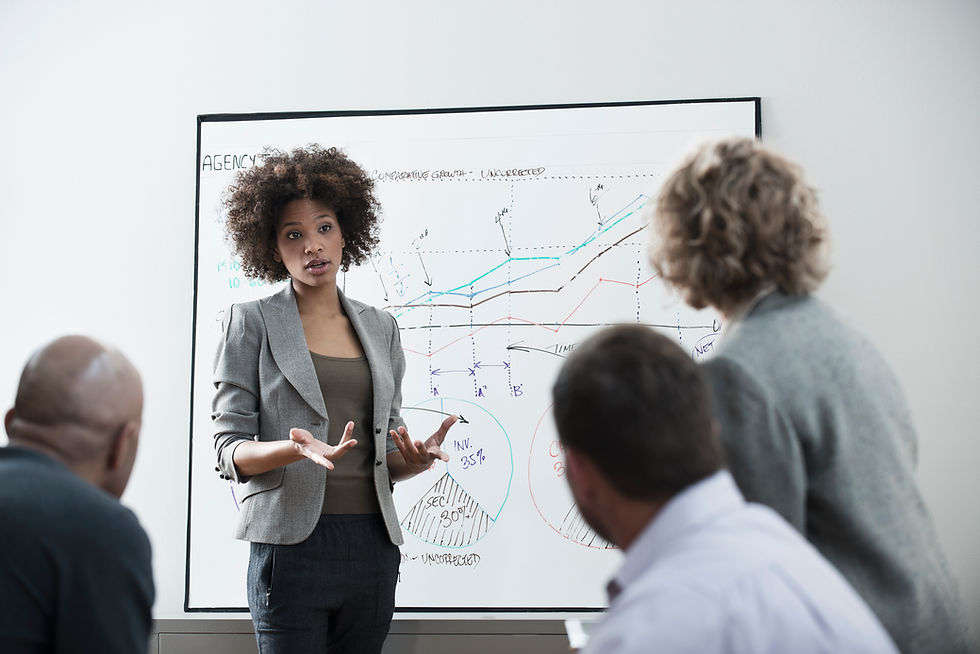
That’s when I realized something critical:
As AI PMs, the real metrics we should be chasing aren’t just technical — they’re human.
The Wake-Up Call
I was leading a product for intelligent document screening. Our model was performant, our devs were proud, and on paper, everything was green. But when we ran post-deployment interviews, a pattern emerged:
“I like the idea… but I still double-check everything.”
That hit me hard. All our work — and the user didn’t trust the output enough to act on it. That’s when I began shifting my perspective from “what is the model doing” to “what is the human doing with the model?”
What I Track Now (And Why)
Today, when I evaluate an AI feature, I still look at model accuracy — but it's not where I stop. I ask:
Are users adopting it — or avoiding it?
Do they trust it enough to take action?
Does it move a business lever that matters to leadership?
For example, on a recent GenAI deployment for pre-authorizing insurance claims, our best insights came not from the F1 score, but from:
The percentage of claims agents who skipped manual review
The rate of AI suggestions accepted without edits
And the speed-to-resolution improvement over the old system
None of these show up in the model training logs — but they define the product’s success.
A Metric I Underestimated (Until I Didn’t)
If there’s one metric I now pay obsessive attention to, it’s this:
Override Rate
How often users ignore, change, or undo the AI’s recommendation. It’s a direct window into trust — something I used to think was intangible.
One time, we saw a 60% override rate in a chatbot’s auto-responses. Instead of tuning the model immediately, we redesigned the UI to better explain “why” a suggestion was made. The override rate dropped to 23% — without a single model tweak.
Lesson: Sometimes, the problem isn’t the model. It’s the message.
Final Thoughts: The Metrics That Tell the Real Story
Being an AI PM means walking a tightrope — balancing model performance with real-world messiness.
Here’s my take:
Accuracy is table stakes.
Trust is the differentiator.
Business outcomes are the true north.
And the best AI PMs? They don’t just monitor dashboards — they listen between the lines. They track how people behave when no one’s watching. That’s where the real metrics live.
So, the next time you ship an AI feature, ask yourself not “how accurate is the model?” — but “how confident is the user?”
Because in the end, the metric that matters most is belief.
Comments